Graph/RAG Frameworks for Accurate Drug Side Effect Retrieval in Large Language Models
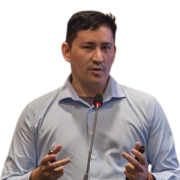
Drug side effects are a leading cause of morbidity and mortality worldwide, highlighting the need for improved detection and analysis methods. Large language models (LLMs) have emerged as powerful tools for generating human-like text. Yet, their knowledge is limited by black-box training data, they are prone to hallucinations, and they often lack domain-specific knowledge. Here, we propose two architectures integrating domain knowledge about drug side effects into a Llama 3-8B Language Model through Retrieval Augmented Generation (RAG) and GraphRAG. Our extensive evaluations on 21,765 associations between 1,106 marketed drugs and 3,903 unique side effect terms show that GraphRAG achieves an accuracy of 99% in drug side effect retrieval. This suggests that our framework could be useful in providing accurate information on drug side effects in the context of Large Language Models.