Standardized Radiomic Image Feature Calculation
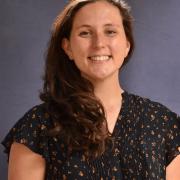
For decades, radiology has largely relied on human-read images, in the form of X-Ray, MRI, and CT. Radiomics, the high throughput calculation of texture features, is a growing field in light of recent advances in computational technology. Standardization needs to occur for these statistics to have significance. There are a number of steps in the computed tomography reconstruction process, such as slice thickness, image kernel, and whether or not filters are applied, that affect the resulting metrics. To start with harmonizing post-reconstruction proceedings, the International Biomarker Standardization Initiative (IBSI) codified a comprehensive set of image feature definitions. This harmonizes post reconstruction proceedings and clarifies the common quantitative language.
Our lab has translated these definitions into both MATLAB and Octave. The 463 image features include eight main subgroups. We verified the accuracy of our algorithm with the given digital phantom provided by the authors of the initiative. After building the tool, its use was investigated in two recently published studies.
The first project sought to contextualize the biomarkers themselves. As the tie between image feature and biological significance is yet to be made, gaining an understanding of the textural implication of the individual statistics is valuable information. Identifying metrics that vary with certain categories, e.g. high disorder, versus the constant metrics between those categorizations, elucidates the behavior of the standardized metrics. This was acheived with the Brodatz standard textures, a database in use for decades to validate a variety of image classification and analysis algorithms. Certain metrics, primarily in the grey level run length matrix (GLRLM), neighborhood grey level dependence matrix (NGLDM), and grey level co- occurence matrix (GLCM) subgroups were found to identify differences across textural types best. As necrotic and tumoral tissues vary in texture relative to normal tissue, identifying abnormal texture is potentially useful.
The second project quantified difference in reconstruction algorithms for computed tomography (CT) scans. As radiology has largely focused on improving image clarity, an unintended consequence of image clarification is corruption of the raw data. The filters and reconstruction algorithms applied make it difficult to compare any calculated metrics from different scans, or even the same scan. A renal scan was reconstructed in the 58 different reconstruction kernels available for that scanner. The 463 image features for this scan were then calculated for five different regions of interest (ROIs). The degree of variation across image kernels was not consistent, and multiple outliers were produced in a handful of image kernels. The quantitative investigation of these acknowledged differences is a necessary precursor for an informed harmonization algorithm.
Ultimately, the goal is widespread use. In order for meaningful comparisons to be made with these statistics, reliable calculation is necessary. Defining the language of comparison is also crucial. By moving towards standardized calculation, the groundwork for future multicenter studies is laid.