Efficient Deep Learning Inferencing on Cloud Kubernetes Clusters using Smart Arm Node Provisioning
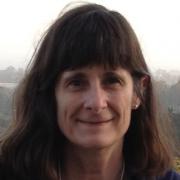
Deep Learning (DL) models are being successfully applied in a variety of fields. Managing DL inferencing at scale for diverse models presents cost and operational complexity challenges.
We have developed an approach to efficient DL inferencing on cloud Kubernetes (K8s) cluster resources, which combines right-sizing the inference resources and the inference compute types, via smart provisioning of Ampere A1 Arm nodes. We have evaluated the benefits of the approach using inference workloads running on auto-scaled TorchServe deployments hosted on Google GKE and Oracle OKE cloud K8s clusters, comparing the cost and operational complexity of right-sizing against two common non-right-sized approaches.
This talk presents these right-sizing results and also includes an additional evaluation on AWS EKS Graviton Arm nodes, to assess how the results are impacted by differences in compute type costs and Arm architectures.